AI in Healthcare: Personalized Medicine and Diagnostics
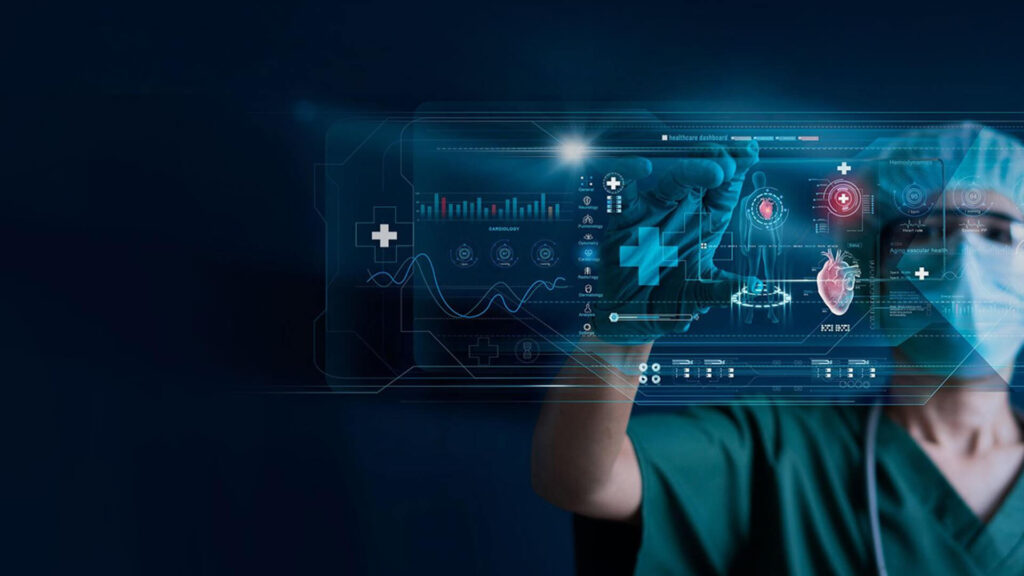
Introduction
Artificial Intelligence (AI) is revolutionizing the healthcare industry, particularly in the realms of personalized medicine and diagnostics. By leveraging advanced algorithms and vast amounts of data, AI enables more precise, efficient, and tailored medical care. This article explores the transformative impact of AI in healthcare, focusing on personalized medicine and diagnostics.
The Role of AI in Personalized Medicine
Personalized medicine refers to medical care designed to cater to the individual characteristics of each patient. This approach contrasts with the traditional one-size-fits-all methodology, offering treatments based on the patient’s genetic profile, lifestyle, and environment.
Key Benefits of AI in Personalized Medicine
- Genomic Analysis: AI algorithms can analyze genetic data to identify mutations and variations linked to specific diseases. This allows for early detection and personalized treatment plans tailored to the genetic makeup of each patient.
- Predictive Analytics: By examining historical health data and lifestyle factors, AI can predict disease risk, helping doctors to intervene early and customize preventive measures.
- Treatment Optimization: AI assists in determining the most effective treatments for patients by analyzing data from clinical trials, patient records, and real-world evidence. This ensures that patients receive the most suitable therapies with minimal side effects.
AI-Powered Diagnostics
AI is also making significant strides in medical diagnostics, enhancing the accuracy and speed of disease detection and diagnosis.
Key Applications of AI in Diagnostics
- Medical Imaging: AI algorithms can interpret medical images (e.g., X-rays, MRIs, CT scans) with high precision, identifying abnormalities that might be missed by human eyes. This leads to quicker and more accurate diagnoses.
- Pathology: AI-powered tools can analyze pathology slides to detect cancerous cells, reducing the workload for pathologists and increasing diagnostic accuracy.
- Early Detection: AI systems can process and analyze large datasets from various diagnostic tests to identify early signs of diseases such as cancer, diabetes, and cardiovascular conditions, facilitating prompt intervention.
Case Studies and Real-World Examples
Case Study 1: IBM Watson for Oncology
IBM Watson uses AI to provide oncologists with evidence-based treatment options. By analyzing medical literature, clinical trial data, and patient records, Watson helps doctors develop personalized cancer treatment plans.
Case Study 2: Google DeepMind and Diabetic Retinopathy
Google’s DeepMind developed an AI system capable of diagnosing diabetic retinopathy with high accuracy from retinal scans. This technology aids in early detection, preventing vision loss in diabetic patients.
Challenges and Ethical Considerations
While AI offers numerous benefits, its integration into healthcare comes with challenges and ethical concerns:
- Data Privacy: Ensuring the privacy and security of patient data is paramount. Robust measures must be in place to protect sensitive information from breaches.
- Bias in AI Algorithms: AI systems can inherit biases present in training data, leading to unequal treatment outcomes. Continuous efforts are required to identify and mitigate these biases.
- Regulatory Hurdles: The adoption of AI in healthcare is subject to regulatory approvals, which can be time-consuming. Clear guidelines and standards are needed to facilitate the safe and effective use of AI technologies.
The Future of AI in Healthcare
The future of AI in healthcare is promising, with ongoing advancements poised to further enhance personalized medicine and diagnostics. Innovations in AI and machine learning will continue to drive the development of more precise, efficient, and patient-centric healthcare solutions.
Conclusion
AI is transforming healthcare by enabling personalized medicine and improving diagnostic accuracy. The benefits of AI-driven genomic analysis, predictive analytics, and optimized treatment plans are revolutionizing patient care. Despite challenges such as data privacy and algorithmic bias, the potential of AI in healthcare is immense. As technology advances, AI will play an increasingly vital role in delivering personalized, efficient, and effective medical care.